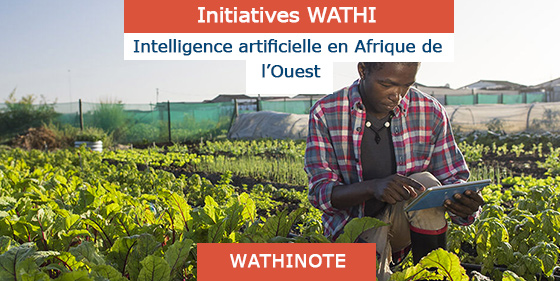
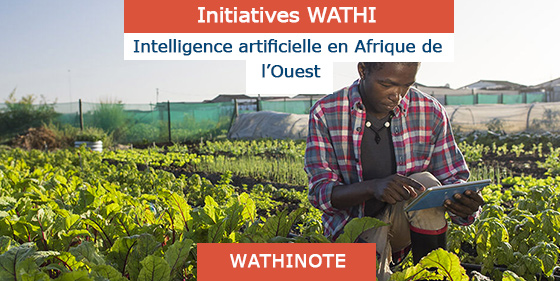
Authors : Matthew M. Young, Justin B. Bullock, Jesse D. Lecy
Type of publication : Academic article
Date of publication : 2019
Advances in the field of artificial intelligence (AI) have profoundly changed the practice of decision making in organizations, thus posing fundamental changes to public administration as well. AI, similar to other information communication technologies (ICT), continues to augment and supplant human discretion within bureaucracies and governance entities.
With equal ease, artificial intelligence can be used for pro-social and equity-enhancing projects or to generate discriminative and regressive outcomes.
As administration tasks shift from humans to machine agents, public managers need tools to anticipate the impact of deploying these technologies and to assess how programs’ costs and benefits might accrue to subpopulations differently.
Public managers and policymakers will be interested in how artificial discretion can improve outcomes from programs that currently rely on human administrative discretion.
We argue that AD [Artificial Discretion] is a governance tool whose optimal use differs according to the specificity of the task complexity of the task environment, that is, the degree of discretion needed to complete the task effectively. Thus, we define artificial discretion (AD) as cases where artificial intelligence is used to augment or automate the exercise of administrative discretion.
Unlike the displacement of low-skill human labor in manufacturing through automation, AI has the potential to target jobs that have typically been immune from automation because they require specialized cognitive skills. Law, for example, lends itself nicely to AI systems because legal databases and natural language processing algorithms can be used to model case law.
Many bureaucratic or managerial tasks in the public and nonprofit sectors have similar characteristics as those in law, creating opportunities to automate specific tasks or wholly replace some public servants. There is no economic rationale for the public sector to favor human labor over machine labor
Uses for Artificial Discretion in Governance
Artificial discretion presents an opportunity to improve on the status quo in government by addressing deficiencies that are ubiquitous in administrative decision making.
These problems include (1) inaccurate predictions on consequential discretionary tasks such as placing a child into foster care or granting a small business loan; (2) inconsistent quality of discretion through variance in accuracy across managers, or variance across time because of decision fatigue or emotional shocks; (3) bias in discretion such as inconsistent citation rates in policing; (4) corruption that results when discretionary tasks are used to gain influence such as granting lucrative government contracts to political allies; and (5) the high labor costs of semi-routine bureaucratic tasks that require some discretion, such as processing applications to administer permits.
AI offers opportunities to improve on each of these dimensions by being more accurate, more consistent in ways that reduce variance and bias, less corruptible by having a stronger relationship between data inputs and decisions, and more cost-effective by reducing labor costs associated with mundane tasks.
Public managers will be interested in three principal uses of artificial intelligence in government: (1) creating structured data from unstructured inputs such as images, sensors, or text; (2) leveraging large and multidimensional data sets to identify patterns, generate new insights, or make accurate predictions; and (3) eliminating human components of administrative tasks through automation.
Artificial discretion presents an opportunity to improve on the status quo in government by addressing deficiencies that are ubiquitous in administrative decision making
Each of these categories of AI applications is potentially valuable for public sector organizations, but not every administrative task can be easily improved by AI. These tasks vary along several dimensions, including the amount, veracity, and completeness of information available to the agent at the time of a decision, the cost, risk, and reward associated with the decision, the amount of goal or value ambiguity, and the stakeholders involved.
In cases where tasks require a relatively low level of discretion, automation is appropriate. The potential benefits include cost-savings, and eliminating situations where low-discretion tasks are dull and repetitive to human agents, leading to low-quality outputs and outcomes. Alternatively, automation can be used when the quality of discretion varies significantly across different human agents. Using AI as a decision-support tool can improve discretion when tasks are less structured, data are scarce, or outcomes are harder to define.
Some tasks are straightforward, narrowly defined, and relatively constrained (e.g., issuing parking tickets), whereas others are informed by procedures but consist of a broader set of possible choices (e.g., law enforcement intervening in a domestic dispute). Many others are highly complex, open-ended, and largely unstructured, especially in the design phases of policies or programs.
The more actors the process requires, the more constrained and interdependent discretion becomes. Thus, tasks can be described by whether they can be implemented at the individual level (micro), the team or intraorganizational level (meso), or the institutional and policy-setting level (macro)
An Evaluation Framework for Artificial Discretion
Artificial discretion presents both opportunities and challenges for the public sector. Potential positive outcomes include higher quality, lower cost, or novel government services, and increased consistency in the exercise of discretion. Possible negative outcomes include the erosion of transparency and accountability, distance from tasks that might nurture learning and innovation, a significant risk of increasing inequality, and increases in the capacity for administrative evil.
Technologies used to improve the exercise of discretion will assumedly have a positive impact on performance. The unit of analysis in this evaluative framework is the decision task (hereafter ‘task’), a discrete instance where an agent uses the discretion afforded them to make a decision. The appropriate counterfactual to artificial discretion is nonaugmented human discretion exercised by a public administrator in the same task environment.
In cases where tasks require a relatively low level of discretion, automation is appropriate. The potential benefits include cost-savings, and eliminating situations where low-discretion tasks are dull and repetitive to human agents, leading to low-quality outputs and outcomes
Artificial discretion enjoys a clear comparative advantage with respect to task scalability and cost. However, the relative quality of artificial versus human discretion is less clear because it requires a balanced assessment of task scalability, task cost, and task quality.
Care is needed to avoid overly preferencing cost reductions. Furthermore, possible applications for AD include tasks that are not currently feasible for governments. Such applications could lead to new programs instead of augmenting existing ones, complicating the cost-savings argument.
Task quality is where artificial discretion’s comparative advantage is both more nuanced and fluid as the technology matures. In some cases, artificial discretion has surpassed human discretion with respect to task quality. For example, AD has replaced human agents for routine image identification tasks such as identifying the amount of money and signatures on handwritten checks, obviating the need for staff to verify the amount manually at the time of deposit.
A well-known problem with administrative discretion is human bias, or cognitive limitations that prohibit an agent from making the correct decision. Of concern—especially in the public sector—is bias that arises from prejudice against individuals based on their innate characteristics, religious beliefs, or other affiliations.
We argue that public sector use of artificial discretion should be evaluated according to the five criteria put forward by Salamon (2002): effectiveness, efficiency, equity, manageability, and political feasibility.
For tasks with lower levels of discretion, artificial discretion will often dominate human discretion in terms of effectiveness. But in a subset of such cases, human agents may not be the appropriate unit of comparison.
Artificial discretion enjoys a clear comparative advantage with respect to task scalability and cost. However, the relative quality of artificial versus human discretion is less clear because it requires a balanced assessment of task scalability, task cost, and task quality
It is currently unclear at what level of discretion overall effectiveness related to the utilization of AD begins to decline and human discretion becomes likely to remain more effective. Organizations should thus tread carefully when experimenting with artificial discretion—especially with respect to its use for automation.
Unlike effectiveness, the efficiency of artificial discretion is not a function of task discretion. The high scalability and low unit and marginal costs of AD make it dominant to human efficiency for any discrete task that it can complete.
Public sector use of artificial discretion carries clear equity concerns for person- and place-based predictive policing. These issues are increasingly apparent as machine-learning systems trained on administrative. However, as with the criterion of effectiveness, properly evaluating artificial discretion’s equity effects requires benchmarking it both against the normative value of maximal equality and AD’s impact relative to alternatives.
A central if not always explicated normative argument for automating public sector tasks is that it represents the ideal form of a rational-technical public administration unfettered by moral hazard. In circumstances where expert system-based automation is not possible, artificial discretion-based automation still affords elected officials increased control by eliminating human bureaucrats.
Even simple implementations of artificial discretion itself, however, create their own manageability challenges. Because it is so easy to scale, AD invites overambitious project or policy design, which can lead to overly or unnecessarily complex administrative systems.
The final criterion of legitimacy and political feasibility is defined as whether the general public and politically active agents deem a tool legitimate (appropriate for public action) and politically feasible (either enough political will exists to implement the tool or not enough political will exists to oppose it).
Exactly what constitutes a legitimate use of artificial discretion has yet to be determined across any level of task discretion or context. That said, the interaction of the previous evaluative criteria with current and future AD applications may make its adoption more or less palatable. In cases where using AD allows for outcomes that are both broadly considered prosocial and simply not possible via human cognition, it is easy to envision AD’s acceptance as legitimate.
Conclusion
Potential applications of artificial discretion in government are hard to predict. We know that low-discretion tasks can be automated, but AI is typically cost-effective when tasks are of high volume, so high fixed costs of new system design can be offset by savings from significant reductions in the marginal costs of tasks.
But in cases of many emergent technologies, the challenge in predicting impact comes from new tasks that are made feasible, not the rationalization of existing tasks.
Les Wathinotes sont soit des résumés de publications sélectionnées par WATHI, conformes aux résumés originaux, soit des versions modifiées des résumés originaux, soit des extraits choisis par WATHI compte tenu de leur pertinence par rapport au thème du Débat. Lorsque les publications et leurs résumés ne sont disponibles qu’en français ou en anglais, WATHI se charge de la traduction des extraits choisis dans l’autre langue. Toutes les Wathinotes renvoient aux publications originales et intégrales qui ne sont pas hébergées par le site de WATHI, et sont destinées à promouvoir la lecture de ces documents, fruit du travail de recherche d’universitaires et d’experts.
The Wathinotes are either original abstracts of publications selected by WATHI, modified original summaries or publication quotes selected for their relevance for the theme of the Debate. When publications and abstracts are only available either in French or in English, the translation is done by WATHI. All the Wathinotes link to the original and integral publications that are not hosted on the WATHI website. WATHI participates to the promotion of these documents that have been written by university professors and experts.