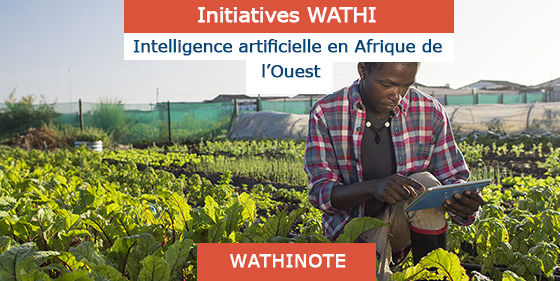
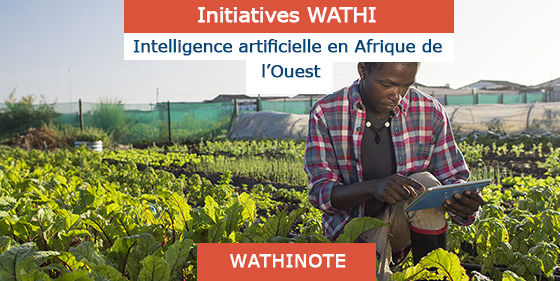
Authors : Kurubaran Ganasegeran and Surajudeen Abiola Abdulrahman
Type of publication : Academic article
Date of publication : 2020
Infectious diseases disrespect national and international borders. They pose substantial threats and serious repercussions to global public health security. Despite coordinated global efforts, containing the “red alert” pandemic of Zika remained a challenge, as both healthcare workers and public health advocates were uncertain about such disastrous contagion causing serious complications including congenital microcephaly in newborns and neurological deficits in adults.
This debacle gave rise to an urgent need to debate the circumstances under which the Zika epidemic has challenged human intelligence behavior and capacity to battle the threat effectively and efficiently. ? Scientists believe that the time has come to institute analytic technologies—such as Artificial Intelligence (AI)—in healthcare to help prevent and resolve such large disease epidemics.
From the healthcare perspective, massive data have been obtained from public health surveillance efforts with the advancement of AI. One major public health field that gained momentum to develop various AI applications for disease prevention was the infectious disease domain. Epidemic modeling and disease-spread simulations form new horizons to understand the effects of citizen behaviors or government health policy measures.
Conceptualizing Artificial Intelligence in Disease Epidemics
Dengue, Chikungunya or Zika, of which these diseases are commonly caused by the vector mosquito. In massive epidemics, elimination of the vector is important, and human cognitive functions can never detect all mosquitos in an outbreak investigation area! However, this can be easily detected through deployment of AI in areas which have loads of mosquito vectors to facilitate control measures.
Applications of AI During Disease Epidemics: Concept Examples
Twitter, a free social-networking micro-blogging service has enabled loads of users to send and read each other’s “tweets (short, 140-character messages).” As important information and geo-political events are embedded within the Twitter stream, researchers now postulate that Twitter users’ reactions may be useful for tracking and forecasting behavior during disease epidemics.
The Zika pandemic between the years of 2015 and 2016 marked the largest known outbreak, reaching a “red-alert” warning of multiple complications requiring global public health interventions. In such exigencies, population health behaviors are important for potential control measures. Daughton and Paul postulated that internet data has been effective to track human health seeking behaviors during disease outbreaks. They used Twitter data between 2015 and 2016 respectively to identify and describe self-disclosures of an individual’s behavior change during disease spread. They combined keyword filtering and ML classifications to identify first-person reactions to Zika.
This finding concludes that applying AI concepts could contribute to better understanding on how public perceives and reacts towards the risks of infectious disease outbreak.
Signorini and colleagues in 2011 analyzed Twitter embedded data for tracking rapid evolvement of public concerns with respect to H1N1 or swine flu, while concurrently measuring actual disease activity.
The results showed that Twitter was useful to measure public interest or concern about health-related events associated with H1N1. These include an observed periodical spikes related to user Twitter activity that were linked to preventive measures (hand-hygiene practices and usage of masks), travel and food consumption behaviors, drug related tweets about specific anti-viral and vaccine uptake. They concluded that Twitter accurately estimated influenza outbreak through AI applications.
The integration of internet data into public health informatics has been regarded as a powerful tool to explore real-time human health-seeking behaviors during disease epidemics. One such popular tool widely utilized is Google Trends, an open tool that provides traffic information regarding trends, patterns and variations of online interests using user-specified keywords and topics over time.
They used Twitter data between 2015 and 2016 respectively to identify and describe self-disclosures of an individual’s behavior change during disease spread. They combined keyword filtering and ML classifications to identify first-person reactions to Zika
Dengue is highly endemic across the South-East Asian countries. Recently, a group of researchers from the Philippines conducted an infodemiology and infoveillance study by using spatio-temporal concepts to explore relationships of weekly Google Dengue Trends (GDT) data from the internet and dengue incidence data from Manila city between 2009 and 2014.
Their findings suggested that weekly temporal GDT patterns were nearly similar to weekly dengue incidence reports. Themes retrieved from dengue-related search queries include: “dengue,” “symptoms and signs of dengue,” “treatment and prevention of dengue,” “mosquito,” and “other diseases.” Most search queries were directed towards manifestations of dengue. The researchers concluded that GDT is a useful component to complement conventional disease surveillance methods.
An unprecedented Ebola contagion that plagued most West African countries in 2014 marked the rise of global public health interest in pandemic preparedness interventions. Millions of Ebola-related internet hit searches were retrieved. With such high fluxes of health-seeking behavior using computers, a group of Italian researchers’ evaluated Google Trends search queries for terms related to “Ebola” outbreak at the global level and across countries where primary cases of Ebola were reported.
Correlations between the total number of Ebola cases registered in all countries and the Google Trends index was relatively high. The researchers concluded that Google Trends data strongly correlated with global epidemiological data. Global agencies could utilize such information to correctly identify outbreaks, and craft appropriate actionable interventions for disease prevention urgently.
Public reactions from Italy 150 K. Ganasegeran and S. A. Abdulrahman toward Chikungunya outbreaks were mined from Google Trends, Google News, Twitter traffics, Wikipedia visits and edits, and PubMed articles to yield a structural equation model. The relationships between overall Chikungunya cases, as well as autochthonous cases and tweet productions were mediated by Chikungunya-related web searches. They concluded that health authorities could be made aware immediately of such phenomenon with the aid of new technologies for collecting public concerns, disseminating awareness and avoiding misleading information.
Malaria constitutes a “red-alert” health threat to the African communities. A group of researchers from Nigeria built an expert system for malaria environmental diagnosis with the aim of providing a decisional support tool for researchers and health policy-makers. The system was able to act as a diagnostic tool to determine the intensity of malarial parasites in designated geographical areas across Africa. The proposed prototype proved useful and cost-effective in curbing malaria spread
Challenges of AI Applications During Disease Epidemics
Whereas AI is gaining increasing popularity and acceptance as a quick fix to the myriad of challenges faced with pandemic preparedness using traditional population-based approaches, it is not without its own limitations. Even in resource-rich settings, there are challenges associated with building and updating the knowledge base of expert systems [29], providing high-quality datasets upon which machine learning algorithms can be premised, and ethical issues associated with data ownership and management.
Correlations between the total number of Ebola cases registered in all countries and the Google Trends index was relatively high. The researchers concluded that Google Trends data strongly correlated with global epidemiological data
More than ever before, public health experts, IT developers and other stakeholders must work together to address concerns related to scalability of AI for healthcare, data integration and interoperability, security, privacy and ethics of aggregated digital data. Finally, the transparency of predictive AI algorithms have been called to question, particularly given their ‘black box’ nature which makes them prone to biases in settings of significant inequalities.
Perhaps, it may be premature to describe AI as the future of healthcare given it is still in its infancy, however, it has become increasingly difficult to not acknowledge the substantial contributions of AI systems to the field of public health medicine. Notwithstanding current challenges with the widespread adoption of AI particularly in resource-limited settings, the use of AI in providing in-depth knowledge on individuals’ health, predicting population health risks and improving pandemic preparedness capabilities is likely to increase substantially in the near future.
Further, the rapidly expanding mobile phone penetrance, developments in cloud computing, substantial investments in health informatics, electronic medical records (EMRs) and mobile health (mHealth) applications, even in resource-constrained settings, holds significant promise for increasing use and scalability of AI applications in improving public health outcomes. Public health policy, practice and research will continue to benefit from the expanding framework of infodemiology and infoveillance in analyzing health information search, communication and publication behavior on the internet.
More than ever before, public health experts, IT developers and other stakeholders must work together to address concerns related to scalability of AI for healthcare, data integration and interoperability, security, privacy and ethics of aggregated digital data
Amazing as the future of AI in healthcare seems, there are significant legal and ethical concerns that need to be addressed in order to pave way for robust implementation and scalability across a variety of socio-cultural, epidemiological, health system and political contexts.
Les Wathinotes sont soit des résumés de publications sélectionnées par WATHI, conformes aux résumés originaux, soit des versions modifiées des résumés originaux, soit des extraits choisis par WATHI compte tenu de leur pertinence par rapport au thème du Débat. Lorsque les publications et leurs résumés ne sont disponibles qu’en français ou en anglais, WATHI se charge de la traduction des extraits choisis dans l’autre langue. Toutes les Wathinotes renvoient aux publications originales et intégrales qui ne sont pas hébergées par le site de WATHI, et sont destinées à promouvoir la lecture de ces documents, fruit du travail de recherche d’universitaires et d’experts.
The Wathinotes are either original abstracts of publications selected by WATHI, modified original summaries or publication quotes selected for their relevance for the theme of the Debate. When publications and abstracts are only available either in French or in English, the translation is done by WATHI. All the Wathinotes link to the original and integral publications that are not hosted on the WATHI website. WATHI participates to the promotion of these documents that have been written by university professors and experts.