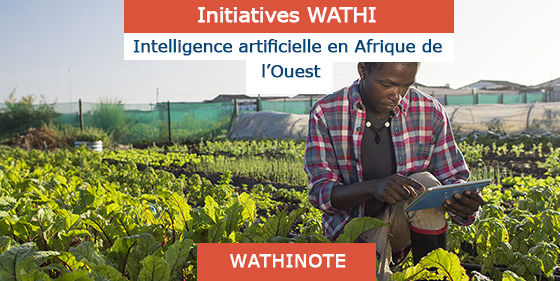
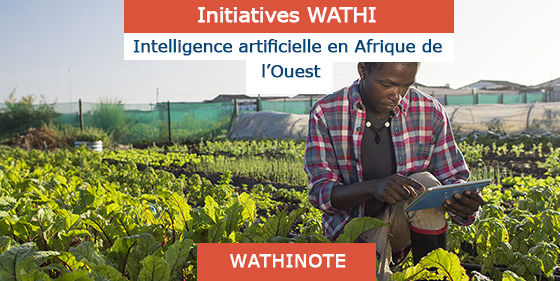
Authors : Nils Köbis, Christopher Starke & Iyad Rahwan
Type of publication : Academic article
Date of publication : 2021
Emerging in various forms, it undermines efficiencies of public institutions, widens gaps in inequalities and thereby hinders the achievement of the UN sustainable development goals. The advancement of digital technologies, in particular artificial intelligence, provide new hope to fight corruption more effectively. Already implemented in several pioneering projects, it can take over anti-corruption tasks like predicting, detecting and disclosing corruption cases.
AI for anti-corruption is not a tool for governments to scrutinize its citizens, but can be a tool for citizens to scrutinize their government. Thus, AI in the fight against corruption evokes much enthusiasm – being praised “as the next frontier in anti-corruption”.
How to harness the potential of AI-ACT
The uniqueness of AI compared to other technologies lies in its autonomous, learning abilities. Instead of a programmer specifying the machine’s course of action for all possible outcomes, AI algorithms can figure out solutions themselves, some of which are unpredictable, even for the human programmers. Many hope that these autonomous learning abilities can contribute to the increasingly data-driven efforts to fight corruption.
In pursuit of more transparency, e-government initiatives, open data programs and citizen-driven crowdsourcing efforts are making ever more data publicly available. According to a long-standing assumption, this growing availability of information will enable citizens to educate and coordinate themselves in the fight against corruption. However, recent policy-oriented research reveals that the mere disclosure of data does not suffice to curb corruption.
These autonomous abilities of AI can be used for anti-corruption efforts adopting top-down or bottom-up approaches. On the one hand, top-down approaches rest on the view that institutions are shaped by laws crafted by political leaders. Hence, top-down anti-corruption efforts seek to bring about change by introducing new laws, regulations and procedures within public administration. AI can be used to assist such approaches.
Bottom-up approaches, on the other hand, view institutions as emerging rather spontaneously through social norms, customs, traditions, beliefs and values within a society. Bottom-up anti-corruption efforts seek to analyze the given cultural and societal context and then identify as well as support existing efforts within the society that seek to reduce corrupt practices. This approach chiefly relies on active civil society organizations and journalists who can play a watchdog role.
AI-ACT present tools for governments (top-down) and, more importantly, citizens (bottom-up) to inspect the government (officials). The unique twist of AI-ACT is that they are tools to tackle abuse of power by those in government. Instead of the government taking the role of big brother watching over the citizens, AI-ACT, in particular when used by bottom-up efforts, allow the public to turn into many little watchdogs keeping the government in check.
Input Data
Powered by Big Data, the success of AI-ACT crucially depend on the quality and size of available input data. A first key question pertains to the source of the data. The emergence of e-government and open government initiatives is rendering many such data sources publicly available. However, large chunks of government data frequently remain undisclosed, unless hackers or whistleblowers expose them, which brings us to the second data source: data leaks. Several high profile cases of data leaks, such as the Panama Papers or more recently the FinCen Files have helped to unveil impactful corrupt cases.
Third, crowdsourced data describes efforts by citizens to expose corruption. One of the most famous examples is the Indian crowdsourcing portal Ipaidabribe.com. On the website, citizens can report cases in which they (were forced to) pay a bribe, tallying up to more than 197,000 of such reports.
Main issue for AI-ACT relates to the quality of the data, captured by the notion of “garbage in, garbage out”. As with all kinds of data, large amounts of digital data also need to be carefully evaluated in terms of whether (1) they entail good proxies for corruption (i.e. validity) and (2) they are a consistent representation of the underlying factor of the measure
Another aspect of data quality are systematic biases. Although algorithms have the aura of being impartial, objective and hence fair, by now ample empirical evidence from various domains documents that ML algorithms can suffer from systematic biases. Algorithms trained on biased data sets, reproduce and further exacerbate the existing biases in the society.
Taken together, AI-ACT are only as good as their input data. Having access to useful data presents the first challenge, one where top-down efforts have an overall advantage over bottom-up efforts that rely on (voluntarily or involuntarily) released data.
Algorithmic Design
A first key feature to consider in the algorithmic design is the accuracy of the predictions. Here, a trade-off occurs between false positive errors and false negative errors. False positive errors describe the wrong classification of innocent individuals as “corrupt”.
Since corruption accusations come with a strong stigma, such errors bear a large cost for those wrongfully accused. Conversely, false negatives come with the cost of leaving actual corrupt cases undetected, which means a non-negligible cost for public institutions or society as a whole.
Taken together, AI-ACT are only as good as their input data. Having access to useful data presents the first challenge, one where top-down efforts have an overall advantage over bottom-up efforts that rely on (voluntarily or involuntarily) released data
Institutional Implementation
When implementing AI-ACT in a top-down manner it is important to be aware of the immense importance of trust towards such technologies. People quickly distrust new technologies if the decision about the implementation happens behind closed doors or if the workings of the algorithms themselves remain hidden from public scrutiny.
Also, bottom-up approaches that use algorithms without careful implementation can similarly backfire. One concrete threat consists of “spamming” citizens with corruption cases, irrespective of whether they are true or not. False accusations disseminated by AI-ACT can have a desensitizing effect on citizens making them care less about the issue. But, also true accusations can have inadvertent ramifications. For instance, empirical evidence suggests that continuous exposure to negative political news can foster cynicism.
Future Outlook: Emerging Questions for Society-in-the-Loop
Input Data – Which data should be off limits?
In the future, new data sources are conceivable. As digital technologies are increasingly embedded in people’s daily lives, human behavior also leaves digital traces that are collected and stored by internet platforms, smartphones, apps, sensors and other devices. Such digital data traces include self-tracking devices, social media communication, geospatial data, browser history and entail contextual data about when, where and how behaviors occur.
People quickly distrust new technologies if the decision about the implementation happens behind closed doors or if the workings of the algorithms themselves remain hidden from public scrutiny
Although potentially helping to fight the dreadful acts of corruption, would people find the use of such data sources justifiable, even if it leads to privacy breaches? Do citizens’ consider the use of data less acceptable when used to predict versus detect corrupt cases? How does the view of the public differ from the assessment of ethicists, policy makers, and IT developers? Further, who should control and be able to use such data?
Algorithmic Design – How to solve the trade-offs?
Increasing accuracy (reducing false positive and false negative errors) can come to the cost of explainability. That is, sophisticated algorithms drawing on large data sets often produce accurate outcomes that however defy simple explanations – rendering the outcome a “black box” model.
Institutional Implementation – How to mobilize and keep citizens involved?
When implementing AI-ACT a first prerequisite, in particular for bottom-up approaches, consists of mobilizing citizens. The outlined digital crowdsourcing efforts hint at the immense potential, but also provide a warning sign about its shortcomings. On the positive side, crowdsourcing tools can mobilize citizens to report a staggering number of corruption cases.
It is indeed a lot to ask from an average citizen to research, analyze and communicate often-intricate corruption schemes. Imagine AI-ACT that alleviate some of the burden. In the future, average citizens could consult an app that does not monitor them but instead allows citizens to monitor their government. Citizens could see within a few seconds how political networks and affiliations might have influenced public decisions.
Conclusion: The importance of gaining empirical answers
One general way to gain answers to outlined questions consists of conducting research that assesses people’s views on AI-ACT. Recent studies using qualitative methods have provided valuable insights into how people respond to algorithmic management, showing that many are averse to such AI managers. Furthermore, large-scale quantitative methods have helped to crowdsource people’s moral preferences about how AI technologies – in this case autonomous vehicles – should behave.
Citizens could see within a few seconds how political networks and affiliations might have influenced public decisions
Using similar approaches can help to involve citizens in the development and adoption of AIACT. Assessing and recognizing their views and preferences about such tools helps to meet the emerging (ethical) dilemmas that such technological innovations bring about. In particular, since AI-ACT is still in its initial phase such a citizen-centered approach can help to shape its future trajectory, so that AI tools can fulfill some of the hopeful aspirations in the fight against corruption.
Les Wathinotes sont soit des résumés de publications sélectionnées par WATHI, conformes aux résumés originaux, soit des versions modifiées des résumés originaux, soit des extraits choisis par WATHI compte tenu de leur pertinence par rapport au thème du Débat. Lorsque les publications et leurs résumés ne sont disponibles qu’en français ou en anglais, WATHI se charge de la traduction des extraits choisis dans l’autre langue. Toutes les Wathinotes renvoient aux publications originales et intégrales qui ne sont pas hébergées par le site de WATHI, et sont destinées à promouvoir la lecture de ces documents, fruit du travail de recherche d’universitaires et d’experts.
The Wathinotes are either original abstracts of publications selected by WATHI, modified original summaries or publication quotes selected for their relevance for the theme of the Debate. When publications and abstracts are only available either in French or in English, the translation is done by WATHI. All the Wathinotes link to the original and integral publications that are not hosted on the WATHI website. WATHI participates to the promotion of these documents that have been written by university professors and experts.