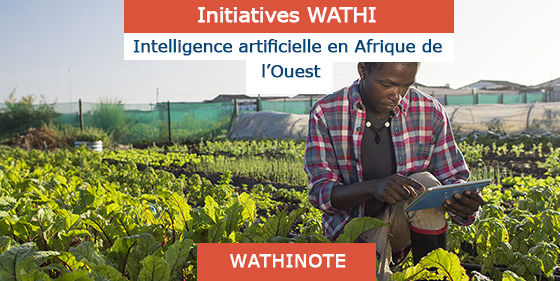
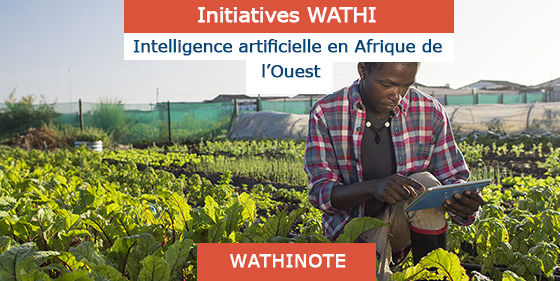
Authors : André Petheram, Walter Pasquarelli & Richard Stirling
Affiliated Organization : oxford insights
Type of publication : Research Report
Date of publication : 2019
As more governments digitise their operations, there is an increased opportunity to develop practical anti-corruption tools based on big data, open data and artificial intelligence. Here, we consider the viability of such tools, in the hope that their implementation would eventually lead to fewer public funds lost to the private accounts of corrupt officials and politicians.
Specifically, we suggest that the conditions are right to test artificial intelligence tools for anti-corruption in a particular group of countries: Argentina, Brazil, Bulgaria, Colombia, Mexico, Paraguay, Romania, Slovakia, Russia, and Ukraine. They score highly in open data rankings, but have high levels of perceived corruption.
Moreover, with an apparent trend towards digitising procurement, often by running government purchasing through online portals, there is a large amount of data that relates to public tenders, contracts and suppliers. This is frequently available openly, providing a valuable resource for anyone seeking to understand the dynamics of corruption through large-scale data analysis. This explains the broad emphasis on procurement in this report.
Using open data for anti-corruption
In recent years open data has become one of the most important methods of enabling transparency. With the increased digitisation of public services and government processes, governments have opportunities to release datasets of progressively higher quality, extent and variety. In addition, anti-corruption authorities may have access to further data that is not publicly available for security, legal or political reasons: government payments data, for example.
Governments are, of course, sensitive about exposing the fine details of their expenditure. In many cases, they may not collect payments data within a central financial management system. However, where available, payments data should be an important target for transparency activists, potentially providing irreplaceably granular information on where public funds go.
Countries in the developing world with high perception of corruption and a low open data ranking, such as Myanmar, Malawi and Zimbabwe, are unlikely to be ready to introduce AI into their anti-corruption efforts
Open data proponents argue that by systematically publishing information on contract amounts, awards and the number of bidders, for example, those contemplating corrupt activities will be discouraged.
Ukraine’s ProZorro e-procurement platform went live in February 2015, one year after the climax of the 2014 Maidan revolution, which saw the corrupt Viktor Yanukovych ousted from the country’s presidency. Before its introduction, Ukraine’s paper-based procurement system lost an estimated $2 billion annually to corruption and other inefficiencies. In spring 2019, the Ukrainian Prime Minister Volodymyr Groysman claimed that since its launch, ProZorro had cut a total of $2.35 billion from procurement.
ProZorro’s impacts appear to support the rationale behind open procurement data. In a 2016 USAID survey of 300 Ukrainian entrepreneurs, 29% of respondents said that they had faced corruption within the ProZorro system as against 54% for the old paper-based system. While still scoring relatively poorly, the country has risen by ten places since 2017 in Transparency International Corruption Perceptions Index.
In January 2018, Digiwhist launched opentender.eu, a platform which allows users to easily examine details of at least 17.5 million EU tenders, dating back to 2003 and with a total value of above €27 billion. Updated twice a year, the platform includes a tender ‘integrity’ filter function, so that tenders with comparatively high corruption risks can be identified.
Opentender’s central innovation is to use data crawling algorithms that systematically work through websites containing open tendering information (such as the EU’s Tenders Electronic Daily), download and structure the data included, and combine this with information taken from other sources about a company’s history and location, and its political connections.
That is, both Opentender and ProZorro demonstrate how big and open data can be translated into user-friendly corruption monitoring tools. To be fully effective, however, such tools must be embedded within a wider system of scrutiny, anti-corruption legislation and effective enforcement on the part of authorities. This way, it will be possible to establish the use cases that prove the utility of open data within anti-corruption. The same is true of any anti-corruption tool based on artificial intelligence.
Artificial intelligence: the next step
In the extent and usability of their data, big and open data portals like Opentender provide starting points for developing anti-corruption tools based on artificial intelligence.
The Digiwhist project described above developed an extensive understanding of corruption ‘red flags’ in public procurement. ‘Red flags’ refer to states of affairs, or inputs, within the procurement process that are correlated with corruption, measured as ‘recurrently awarding contracts to a pre-selected company.’ The Digiwhist team isolated 14 inputs as ‘significant and substantial predictors’ of corruption. These included: procurements with only one bidder, contracts being changed once a project has started, and the price of the tender documents.
There are, of course, many possible barriers to successful development and implementation of an anti-corruption machine learning model, including: the absence or non-availability of extensive and high-quality data; authorities’ refusal to sanction such widespread monitoring; and, a poor enforcement environment.
As these examples demonstrate, and building on substantial developments in both big and open data, using machine learning tools to combat corruption is not only viable, but highly likely to enter regular use in multiple countries in coming years
As this suggests, not every country will have the data available to make use of AI in this way. We might use the Global Open Data Index to indicate how much data is potentially available to AI developers working within anti-corruption. This would suggest that countries in the developing world with high perception of corruption and a low open data ranking, such as Myanmar, Malawi and Zimbabwe, are unlikely to be ready to introduce AI into their anti-corruption efforts.
Conclusion
the World Bank is working with Microsoft to develop a machine learning tool that detects anomalies in procurement, combining tendering data with beneficial ownership information. The results are yet to be seen. Another example comes from Spain, where researchers developed a neural network with the capacity to predict that corruption would emerge within certain regions three years ahead of the actual case. Their model suggested that sustained economic growth and ‘an increase in real estate prices’, among other things, predicted cases of corruption. However, the neural network does not seem to be in current use within anti-corruption enforcement.
As these examples demonstrate, and building on substantial developments in both big and open data, using machine learning tools to combat corruption is not only viable, but highly likely to enter regular use in multiple countries in coming years.
Further research into data, artificial intelligence and anti-corruption should move beyond issues of what datasets and algorithms are most useful. The success of AI in fighting corruption will depend on solving problems that have long occupied government transparency advocates. Who is empowered to introduce and sustain reform in government? How do you encourage civil servants to properly value the collection and release of relevant data? These are problems of authority, communication, relationships and power. Answering them will require a deft understanding of government and of human nature, but the rewards will be large.
Les Wathinotes sont soit des résumés de publications sélectionnées par WATHI, conformes aux résumés originaux, soit des versions modifiées des résumés originaux, soit des extraits choisis par WATHI compte tenu de leur pertinence par rapport au thème du Débat. Lorsque les publications et leurs résumés ne sont disponibles qu’en français ou en anglais, WATHI se charge de la traduction des extraits choisis dans l’autre langue. Toutes les Wathinotes renvoient aux publications originales et intégrales qui ne sont pas hébergées par le site de WATHI, et sont destinées à promouvoir la lecture de ces documents, fruit du travail de recherche d’universitaires et d’experts.
The Wathinotes are either original abstracts of publications selected by WATHI, modified original summaries or publication quotes selected for their relevance for the theme of the Debate. When publications and abstracts are only available either in French or in English, the translation is done by WATHI. All the Wathinotes link to the original and integral publications that are not hosted on the WATHI website. WATHI participates to the promotion of these documents that have been written by university professors and experts.