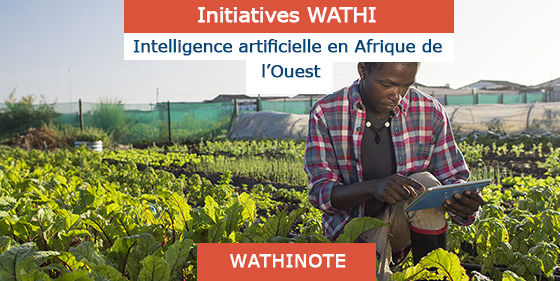
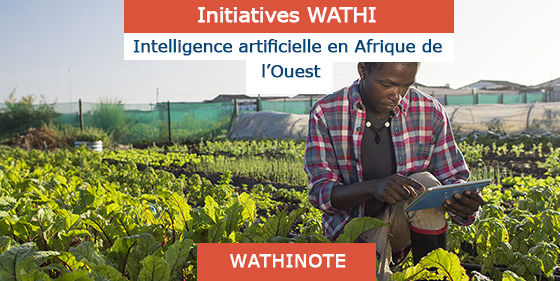
Authors : Thomas Davenport & Ravi Kalakota
Type of publication : Academic article
Date of publication : 2019
Artificial intelligence (AI) and related technologies are increasingly prevalent in business and society, and are beginning to be applied to healthcare. These technologies have the potential to transform many aspects of patient care, as well as administrative processes within provider, payer and pharmaceutical organisations.
There are already a number of research studies suggesting that AI can perform as well as or better than humans at key healthcare tasks, such as diagnosing disease. Today, algorithms are already outperforming radiologists at spotting malignant tumours, and guiding researchers in how to construct cohorts for costly clinical trials. However, for a variety of reasons, we believe that it will be many years before AI replaces humans for broad medical process domains.
Types of AI of relevance to healthcare
In healthcare, the most common application of traditional machine learning is precision medicine – predicting what treatment protocols are likely to succeed on a patient based on various patient attributes and the treatment context. The great majority of machine learning and precision medicine applications require a training dataset for which the outcome variable (eg onset of disease) is known; this is called supervised learning.
The most complex forms of machine learning involve deep learning , or neural network models with many levels of features or variables that predict outcomes. There may be thousands of hidden features in such models, which are uncovered by the faster processing of today’s graphics processing units and cloud architectures. A common application of deep learning in healthcare is recognition of potentially cancerous lesions in radiology images.
Making sense of human language has been a goal of AI researchers since the 1950s. This field, NLP, includes applications such as speech recognition, text analysis, translation and other goals related to language.
In healthcare, the dominant applications of NLP involve the creation, understanding and classification of clinical documentation and published research. NLP systems can analyse unstructured clinical notes on patients, prepare reports (eg on radiology examinations), transcribe patient interactions and conduct conversational AI.
Today, algorithms are already outperforming radiologists at spotting malignant tumours, and guiding researchers in how to construct cohorts for costly clinical trials. However, for a variety of reasons, we believe that it will be many years before AI replaces humans for broad medical process domains
Expert systems require human experts and knowledge engineers to construct a series of rules in a particular knowledge domain. They work well up to a point and are easy to understand. However, when the number of rules is large (usually over several thousand) and the rules begin to conflict with each other, they tend to break down.
Physical robots are well known by this point, given that more than 200,000 industrial robots are installed each year around the world. They perform pre-defined tasks like lifting, repositioning, welding or assembling objects in places like factories and warehouses, and delivering supplies in hospitals. More recently, robots have become more collaborative with humans and are more easily trained by moving them through a desired task.
Surgical robots, initially approved in the USA in 2000, provide ‘superpowers’ to surgeons, improving their ability to see, create precise and minimally invasive incisions, stitch wounds and so forth. Important decisions are still made by human surgeons, however.
Robotic process automation (RPA) doesn’t really involve robots – only computer programs on servers. It relies on a combination of workflow, business rules and ‘presentation layer’ integration with information systems to act like a semi-intelligent user of the systems. In healthcare, they are used for repetitive tasks like prior authorisation, updating patient records or billing. When combined with other technologies like image recognition, they can be used to extract data from, for example, faxed images in order to input it into transactional systems.
Diagnosis and treatment applications
Diagnosis and treatment of disease has been a focus of AI since at least the 1970s, when MYCIN was developed at Stanford for diagnosing blood-borne bacterial infections. This and other early rule-based systems showed promise for accurately diagnosing and treating disease, but were not adopted for clinical practice.
More recently, IBM’s Watson has received considerable attention in the media for its focus on precision medicine, particularly cancer diagnosis and treatment. Watson employs a combination of machine learning and NLP capabilities. However, early enthusiasm for this application of the technology has faded as customers realised the difficulty of teaching Watson how to address particular types of cancer and of integrating Watson into care processes and systems.
These rule-based clinical decision support systems are difficult to maintain as medical knowledge changes and are often not able to handle the explosion of data and knowledge based on genomic, proteomic, metabolic and other ‘omic-based’ approaches to care. This situation is beginning to change, but it is mostly present in research labs and in tech firms, rather than in clinical practice.
There are also several firms that focus specifically on diagnosis and treatment recommendations for certain cancers based on their genetic profiles. Since many cancers have a genetic basis, human clinicians have found it increasingly complex to understand all genetic variants of cancer and their response to new drugs and protocols.
But whether rules-based or algorithmic in nature, AI-based diagnosis and treatment recommendations are sometimes challenging to embed in clinical workflows and EHR systems. Such integration issues have probably been a greater barrier to broad implementation of AI than any inability to provide accurate and effective recommendations; and many AI-based capabilities for diagnosis and treatment from tech firms are standalone in nature or address only a single aspect of care.
Patient engagement and adherence applications
Providers and hospitals often use their clinical expertise to develop a plan of care that they know will improve a chronic or acute patient’s health. However, that often doesn’t matter if the patient fails to make the behavioural adjustment necessary, eg losing weight, scheduling a follow-up visit, filling prescriptions or complying with a treatment plan.
If deeper involvement by patients results in better health outcomes, can AI-based capabilities be effective in personalising and contextualising care? There is growing emphasis on using machine learning and business rules engines to drive nuanced interventions along the care continuum. Messaging alerts and relevant, targeted content that provoke actions at moments that matter is a promising field in research.
Administrative applications
There are also a great many administrative applications in healthcare. The use of AI is somewhat less potentially revolutionary in this domain as compared to patient care, but it can provide substantial efficiencies. These are needed in healthcare because, for example, the average US nurse spends 25% of work time on regulatory and administrative activities. The technology that is most likely to be relevant to this objective is RPA. It can be used for a variety of applications in healthcare, including claims processing, clinical documentation, revenue cycle management and medical records management.
Implications for the healthcare workforce
There has been considerable attention to the concern that AI will lead to automation of jobs and substantial displacement of the workforce.
To our knowledge thus far there have been no jobs eliminated by AI in health care. The limited incursion of AI into the industry thus far, and the difficulty of integrating AI into clinical workflows and EHR systems, have been somewhat responsible for the lack of job impact. It seems likely that the healthcare jobs most likely to be automated would be those that involve dealing with digital information, radiology and pathology for example, rather than those with direct patient contact.
If deeper involvement by patients results in better health outcomes, can AI-based capabilities be effective in personalising and contextualising care?
Ethical implications
Mistakes will undoubtedly be made by AI systems in patient diagnosis and treatment and it may be difficult to establish accountability for them. There are also likely to be incidents in which patients receive medical information from AI systems that they would prefer to receive from an empathetic clinician. Machine learning systems in healthcare may also be subject to algorithmic bias, perhaps predicting greater likelihood of disease on the basis of gender or race when those are not actually causal factors.
We are likely to encounter many ethical, medical, occupational and technological changes with AI in healthcare. It is important that healthcare institutions, as well as governmental and regulatory bodies, establish structures to monitor key issues, react in a responsible manner and establish governance mechanisms to limit negative implications. This is one of the more powerful and consequential technologies to impact human societies, so it will require continuous attention and thoughtful policy for many years.
The future of AI in healthcare
We believe that AI has an important role to play in the healthcare offerings of the future. In the form of machine learning, it is the primary capability behind the development of precision medicine, widely agreed to be a sorely needed advance in care. Although early efforts at providing diagnosis and treatment recommendations have proven challenging, we expect that AI will ultimately master that domain as well.
It seems likely that the healthcare jobs most likely to be automated would be those that involve dealing with digital information, radiology and pathology for example, rather than those with direct patient contact
The greatest challenge to AI in these healthcare domains is not whether the technologies will be capable enough to be useful, but rather ensuring their adoption in daily clinical practice. For widespread adoption to take place, AI systems must be approved by regulators, integrated with EHR systems, standardised to a sufficient degree that similar products work in a similar fashion, taught to clinicians, paid for by public or private payer organisations and updated over time in the field.
It also seems increasingly clear that AI systems will not replace human clinicians on a large scale, but rather will augment their efforts to care for patients.
Les Wathinotes sont soit des résumés de publications sélectionnées par WATHI, conformes aux résumés originaux, soit des versions modifiées des résumés originaux, soit des extraits choisis par WATHI compte tenu de leur pertinence par rapport au thème du Débat. Lorsque les publications et leurs résumés ne sont disponibles qu’en français ou en anglais, WATHI se charge de la traduction des extraits choisis dans l’autre langue. Toutes les Wathinotes renvoient aux publications originales et intégrales qui ne sont pas hébergées par le site de WATHI, et sont destinées à promouvoir la lecture de ces documents, fruit du travail de recherche d’universitaires et d’experts.
The Wathinotes are either original abstracts of publications selected by WATHI, modified original summaries or publication quotes selected for their relevance for the theme of the Debate. When publications and abstracts are only available either in French or in English, the translation is done by WATHI. All the Wathinotes link to the original and integral publications that are not hosted on the WATHI website. WATHI participates to the promotion of these documents that have been written by university professors and experts.