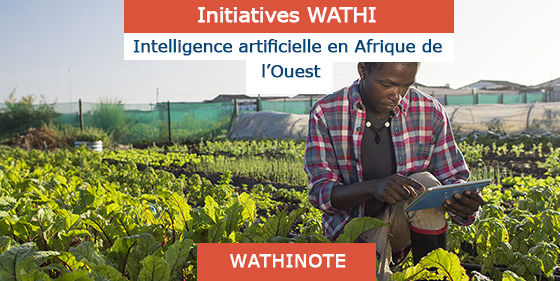
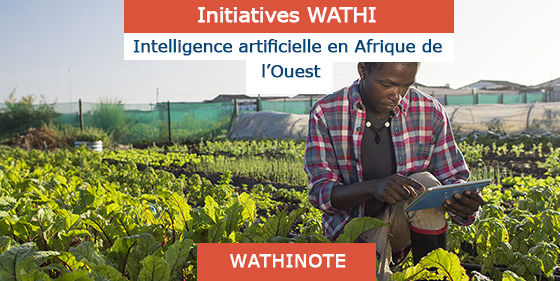
Author : Timnit Gebru
Affiliated organization : MIT Technology review
Type of publication : Interview
Date of publication : Février 2018
Artificial intelligence is an increasingly seamless part of our everyday lives, present in everything from web searches to social media to home assistants like Alexa. But what do we do if this massively important technology is unintentionally, but fundamentally, biased? And what do we do if this massively important field includes almost no black researchers? Timnit Gebru is tackling these questions as part of Microsoft’s Fairness, Accountability, Transparency, and Ethics in AI group, which she joined last summer. She also cofounded the Black in AI event at the Neural Information Processing Systems (NIPS) conference in 2017 and was on the steering committee for the first Fairness and Transparency conference in February.
How does the lack of diversity distort artificial intelligence and specifically computer vision?
There is a bias to what kinds of problems we think are important, what kinds of research we think are important, and where we think AI should go. If we don’t have diversity in our set of researchers, we are not going to address problems that are faced by the majority of people in the world. When problems don’t affect us, we don’t think they’re that important, and we might not even know what these problems are, because we’re not interacting with the people who are experiencing them.
Are there ways to counteract bias in systems?
We are in a diversity crisis for AI. In addition to having technical conversations, conversations about law, conversations about ethics, we need to have conversations about diversity in AI. We need all sorts of diversity in AI. And this needs to be treated as something that’s extremely urgent.
Something I’m really passionate about and I’m working on right now is to figure out how to encourage companies to give more information to users or even researchers. They should have recommended usage, what the pitfalls are, how biased the data set is, etc. So that when I’m a startup and I’m just taking your off-the-shelf data set or off-the-shelf model and incorporating it into whatever I’m doing, at least I have some knowledge of what kinds of pitfalls there may be.
If we don’t have diversity in our set of researchers, we are not going to address problems that are faced by the majority of people in the world. When problems don’t affect us, we don’t think they’re that important, and we might not even know what these problems are, because we’re not interacting with the people who are experiencing them
AI is just now starting to be baked into the mainstream, into a product everywhere, so we’re at a precipice where we really need some sort of conversation around standardization and usage.
What issues are you hoping to address with this first Fairness and Transparency conference?
This is really the first conference that is addressing the issues of fairness, accountability, ethics, and transparency in AI. It’s really important to have the stand-alone conference because it needs to be worked on by people from many disciplines who talk to each other.
Machine-learning people on their own cannot solve this problem. There are issues of transparency; there are issues of how the laws should be updated. If you’re going to talk about bias in health care, you want to talk to [health-care professionals] about where the potential biases could be, and then you can think about how to have a machine-learning-based solution.
What has been your experience working in AI?
It’s not easy. I love my job. I love the research that I work on. I love the field. I cannot imagine what else I would do in that respect. That being said, it’s very difficult to be a black woman in this field. When I started Black in AI, I started it with a couple of my friends.
What really just made it accelerate was [in 2016] when I went to NIPS and someone was saying there were an estimated 8,500 people. I counted six black people. I was literally panicking. That’s the only way I can describe how I felt.
At the same time, I also saw a lot of rhetoric about diversity and how a lot of companies think it’s important. And I saw a mismatch between the rhetoric and action. Because six black people out of 8,500—that’s a ridiculous number, right? That is almost zero percent. I was like, “We have to do something now.” I want to give a call to action to people who believe diversity is important. Because it is an emergency, and we have to do something about it now.
Les Wathinotes sont soit des résumés de publications sélectionnées par WATHI, conformes aux résumés originaux, soit des versions modifiées des résumés originaux, soit des extraits choisis par WATHI compte tenu de leur pertinence par rapport au thème du Débat. Lorsque les publications et leurs résumés ne sont disponibles qu’en français ou en anglais, WATHI se charge de la traduction des extraits choisis dans l’autre langue. Toutes les Wathinotes renvoient aux publications originales et intégrales qui ne sont pas hébergées par le site de WATHI, et sont destinées à promouvoir la lecture de ces documents, fruit du travail de recherche d’universitaires et d’experts.
The Wathinotes are either original abstracts of publications selected by WATHI, modified original summaries or publication quotes selected for their relevance for the theme of the Debate. When publications and abstracts are only available either in French or in English, the translation is done by WATHI. All the Wathinotes link to the original and integral publications that are not hosted on the WATHI website. WATHI participates to the promotion of these documents that have been written by university professors and experts.